Table Of Content
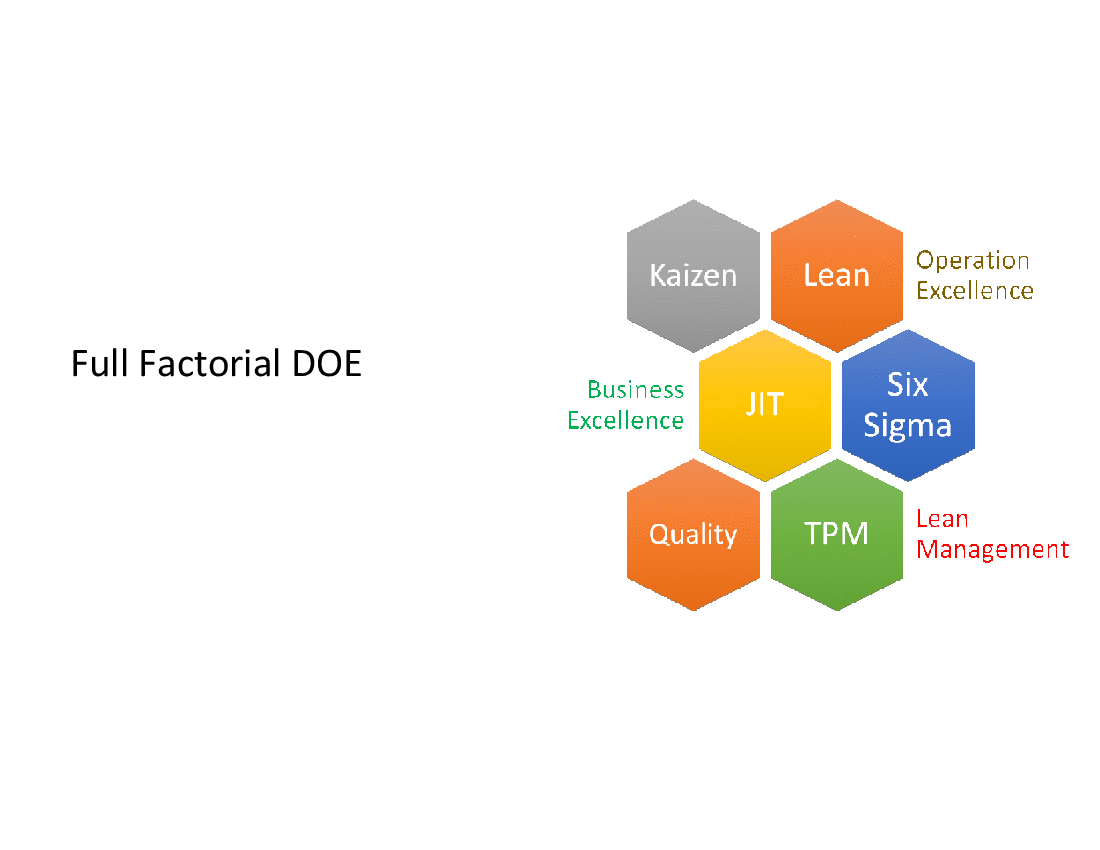
DOE is about creating an entity of experiments that work together to map an interesting experimental region. So with DOE we can prepare a set of experiments that are optimally placed to bring back as much information as possible about how the factors are influencing the responses. These four points can be optimally supplemented by a couple of points representing the variation in the interior part of the experimental design. Next, we evaluate what will happen when we fix the volume at 550 ml (the optimal level) and start to change the second factor. In this second experimental series, the pH is changed from 2.5 to 5.0 and you can see the measured yields.
Factorial Design of Experiments
Ultimately, assessing a particular fractional factorial is about deciding whether you can determine any of the particular higher order effects that you think might be interesting. So, the design doesn’t include the high-order interactions with each other, which drastically reduces run numbers. Though you’ll find that DOE software will often prevent you from making certain choices that your design does not permit. Robustness is a concept that enters into statistics at several points. At the analysis, stage robustness refers to a technique that isn't overly influenced by bad data. Even if there is an outlier or bad data you still want to get the right answer.
Basics to Complex Models
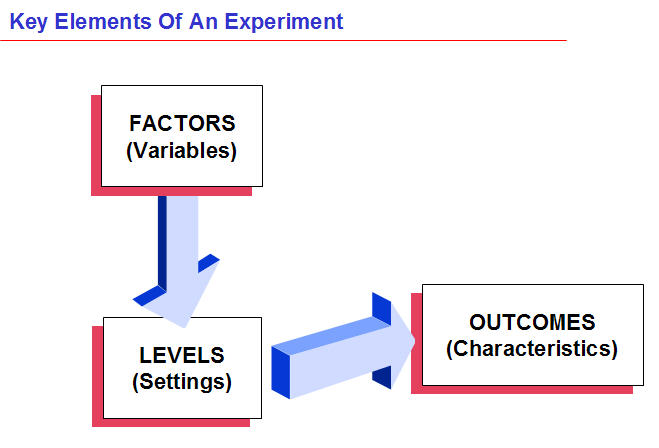
We change the experimental factors and measure the response outcome, which in this case, is the yield of the desired product. Using the COST approach, we can vary just one of the factors at time to see what affect it has on the yield. [This blog was a favorite last year, so we thought you'd like to see it again.
Advanced Complex Mixed Factors
The same is true for intervening variables (a variable in between the supposed cause (X) and the effect (Y)), and anteceding variables (a variable prior to the supposed cause (X) that is the true cause). When a third variable is involved and has not been controlled for, the relation is said to be a zero order relationship. In most practical applications of experimental research designs there are several causes (X1, X2, X3). In most designs, only one of these causes is manipulated at a time. Together, these principles and ethical considerations create a framework for DoE that is robust, respectful, and reflective of the highest ideals of scientific inquiry. They ensure that experiments designed are technically sound, ethically grounded, and philosophically aligned with pursuing a deeper understanding of the world.
Replication, the repetition of the experiment under the same conditions, is vital for assessing the consistency of the results. It enhances the experiment’s reliability, ensuring that the findings are not anomalies but reflect an actual effect. Replication reinforces the integrity of the scientific method, allowing researchers to confidently attribute observed effects to the experimental conditions rather than to random variation. Truth in measurement is the cornerstone, demanding accuracy and reliability in data collection and analysis. This principle challenges researchers to maintain rigor in their methods, ensuring that the insights gleaned reflect reality, untainted by bias or error. The key concept behind this methodology is that there is a relationship between the factors affecting the response.
Feature article – An alternative to DoE - Speciality Chemicals Magazine
Feature article – An alternative to DoE.
Posted: Tue, 19 Dec 2023 08:00:00 GMT [source]
The same problems of having “low resolution” will apply to your DOE design. As when it comes to fitting a model to your data, if your DOE has a really low resolution, you won’t be able to tell the difference between effect a) and effect b). Whereas if you have a “high resolution”, distinguishing between one effect and another is easy. In short, resolution is all about assessing your design by how well you can tell different effects apart. But when you’re new to DOE, there’s nothing worse than having too many choices.
So, if you used a 2-level full factorial design during the refinement and iteration stage, you only need to add the axial points and replicated center points to achieve an RSM design. This website is using a security service to protect itself from online attacks. The action you just performed triggered the security solution. There are several actions that could trigger this block including submitting a certain word or phrase, a SQL command or malformed data. The textbook we are using brings an engineering perspective to the design of experiments. We will bring in other contexts and examples from other fields of study including agriculture (where much of the early research was done) education and nutrition.
Discover content
But taking them both at the same time can cause an interaction effect that can be deadly. During your experiment, you will have your experimental factors as well as other environmental factors around you that you aren’t interested in testing. You will need to control those to reduce the noise and contamination that might occur (which would reduce the value of your DOE). In a conjoint analysis DOE, you would create mockups of the various combinations of variables. A sample of customers were selected and shown the different mockups.
One-Way/Single Factor Analysis of Variance, ANOVA
ISixSigma defined it as determining the “cause and effect relationships” of factors. Therefore, a complete experimental plan consists of the combination of factors used to evaluate their effects on the response. Design of Experiments (DOE) is a systematic method used in applied statistics to evaluate the many possible alternatives in one or more design variables. It allows the manipulation of various input variables (factors) to determine what effect they could have in order to get the desired output (responses) or improve on the result.
DOE lets you balance trade-offs, such as what conditions produce the most cost-effective way to achieve the highest yield of strawberries. The responses we are looking for in this experiment are the yield, the weight, and the taste of the strawberries. You may decide you want a high yield of the tastiest strawberries.
After analyzing all of your main effects and interactions, you will be able to determine what your settings should be for your factors or variables. Only when this is done is it possible to certify with high probability that the reason for the differences in the outcome variables are caused by the different conditions. Therefore, researchers should choose the experimental design over other design types whenever possible. However, the nature of the independent variable does not always allow for manipulation.
].Whether you work in engineering, R&D, or a science lab, understanding the basics of experimental design can help you achieve more statistically optimal results from your experiments or improve your output quality. In reality, there are nuances to the different types of RSM designs. Though some types of RSM designs can be thought of as full factorial designs across 2 levels for each factor, with center and axial points to sample additional levels without needing to do a full factorial across all levels. Full factorial designs are often most appropriate when screening has identified a few important factors to optimize, or when using liquid handling automation affording an increase in throughput. Response Surface Methodology (RSM) is an advanced set of techniques for modeling and analyzing problems in which several variables influence a response of interest. RSM is designed to optimize the response, identify the relationship between variables, and find the conditions that maximize or minimize the response value.
This section reviews notable statistical software packages that support DoE, highlighting features that enhance the research process from design to data visualization. Completely Randomized Design (CRD) is the simplest form of experimental design, where treatments are randomly assigned to experimental units. This design is most effective when dealing with a homogeneous population or when the experiment is conducted under controlled conditions, minimizing the variability among experimental units.
Our goal is usually to find out something about a treatment factor (or a factor of primary interest), but in addition to this, we want to include any blocking factors that will explain variation. When factors or levels increase, full factorial designs can become infeasible—even in sophisticated, high-capacity facilities. You can use fractional factorial designs when you have a large number of factors to screen, or where resources are limited. Some efficient designs for estimating several main effects were found independently and in near succession by Raj Chandra Bose and K.
No comments:
Post a Comment